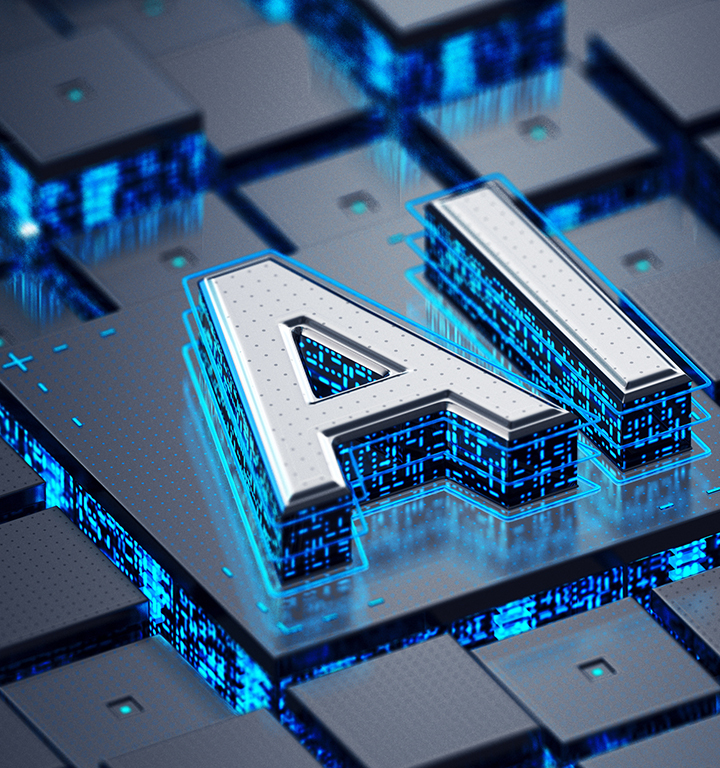
View Expert Insights
February • 27 • 2023
AI in Healthcare: Emerging Opportunities and Risks
Article
Summary
AI in healthcare may reduce spending and improve patient outcomes, but challenges in the technology and adoption require thoughtful risk management.
Artificial intelligence is transforming society. Whether you’re using Netflix’s personalized recommendations to select your next movie or using WAZE to navigate the fastest route, AI-powered advancements seem to be everywhere – including in healthcare. Although AI in healthcare offers exciting opportunities, the technology also has risks. Early adopters must proceed with a full understanding of both the opportunities and the potential exposures.
A 2021 report from Sage Growth Partners found that 90% of healthcare executives had an AI or automation strategy in place – up from 53% in 2019. Furthermore, 76% of respondents said automation has become more important because it can help them recuperate faster by cutting wasteful spending and improving efficiency.
AI could reduce spending in many ways. For example, the technology could help reduce no-shows by identifying patients who might need a ride and providing guidance. Speech recognition technology could improve documentation efficiency and help prevent burnout. In addition, implementation in billing and coding could improve workflows.
There are countless studies that demonstrate the potential impact of AI in healthcare, including:
The above examples make it clear that AI is worth pursuing. The potential benefits for healthcare providers, administrators, and patients are too great to ignore. However, as with every new technology, there are barriers and potential exposures that include:
Accuracy is a key concern, but other issues could also impede AI adoption.
The potential of AI is tremendous, but healthcare leaders need to proceed with caution. Regardless of the type of AI application in question, recommended best practices include:
This article was based in part on a Coverys presentation “Risks and Legal Aspects of Artificial Intelligence in Healthcare,” presented by Matthew P. Keris, Esq., and Judy Recker, MHA, RPh, CPHQ, CPHRM.
Copyrighted. No legal or medical advice intended. This post includes general risk management guidelines. Such materials are for informational purposes only and may not reflect the most current legal or medical developments. These informational materials are not intended, and must not be taken, as legal or medical advice on any particular set of facts or circumstances.
How AI Could Improve Healthcare Spending
A 2021 report from Sage Growth Partners found that 90% of healthcare executives had an AI or automation strategy in place – up from 53% in 2019. Furthermore, 76% of respondents said automation has become more important because it can help them recuperate faster by cutting wasteful spending and improving efficiency.AI could reduce spending in many ways. For example, the technology could help reduce no-shows by identifying patients who might need a ride and providing guidance. Speech recognition technology could improve documentation efficiency and help prevent burnout. In addition, implementation in billing and coding could improve workflows.
The Potential Impact of AI on Patient Outcomes
There are countless studies that demonstrate the potential impact of AI in healthcare, including:
- Detecting cancer. The Massachusetts Institute of Technology says an AI tool can help detect melanoma, and a paper published in npj breast cancer outlines how AI-based tools can support cancer detection and classification in breast biopsies.
- Diagnosing other conditions. Google researchers are using AI to identify diabetic retinopathy, and the University of Florida Department of Surgery says researchers used AI to identify a patient’s likelihood of developing sepsis within 12 hours of hospital admission.
- Informing treatment decisions. Geisinger researchers say an algorithm that uses echocardiogram videos of the heart can outperform other predictors of mortality. AI has also been used to help physicians diagnose and make treatment decisions about intracranial hemorrhage (ICH). A study published in npj digital medicine found that machine learning algorithms could automatically analyze computed tomography (CT) of the head, prioritize radiology worklists, and reduce time to diagnosis of ICH.
- Reducing bias. A team of researchers at Stanford University found that an algorithmic approach could help eliminate bias. Using AI to measure severe knee pain revealed that standard radiographic measures may overlook certain features that cause pain and disproportionately impact minority and low-income populations.
Challenges in AI Adoption
The above examples make it clear that AI is worth pursuing. The potential benefits for healthcare providers, administrators, and patients are too great to ignore. However, as with every new technology, there are barriers and potential exposures that include:
- Inaccuracies and misdiagnoses if AI applications are built on incomplete data. If electronic health records omit key details or if speech recognition tools misinterpret speech and insert incorrect information into a patient’s record, patient outcomes could be adversely affected.
- Flawed tools that could harm patients. According to mHealth Intelligence, the FDA ordered the recall of a diabetes management app because a bug could cause the app to prompt users to inject the wrong amount of insulin. Such miscalculations could prove fatal.
- Results that initially look good may not be as promising as they first appear. Researchers at the Icahn School of Medicine at Mount Sinai wanted to assess how AI models identified pneumonia in chest X-rays from three medical institutions. The tool was significantly less successful in diagnosing pneumonia in X-rays that came from outside the original health system, indicating the tool “cheated” by using information related to the prevalence of pneumonia at the original training institution.
- AI’s vulnerability to cyberattacks. According to Medical Xpress, University of Pittsburgh researchers showed that falsified mammogram images could fool AI, possibly opening the door to cyberattacks.
Other Concerns Surrounding AI in Healthcare
Accuracy is a key concern, but other issues could also impede AI adoption.
- Integration: If an AI model cannot fit into a company’s existing IT infrastructure, adoption may not occur.
- The Black Box: An article published in JAMA notes that unlike traditional clinical decision support software, AI may provide recommendations without underlying reasons.
- Regulatory Approvals: According to Pew Trusts, research indicates that most AI-based medical devices that are considered to be moderate to high risk receive FDA authorization via 510(k) clearance, which requires the manufacturer to demonstrate that the device is substantially equivalent to a device already on the market. There is some concern that this process does not provide thorough and comprehensive review. An improved FDA regulatory framework may be needed to ensure new device safety and effectiveness without causing unreasonable delays.
- Lack of Clinical Trials: Randomized clinical trials are the classic measure of clinical benefit. However, randomized clinical trials are rare with AI. A 2021 study on the development and validation pathways of artificial intelligence tools evaluated in randomized clinical trials by BMJ Health Care Inform found that rigorous evaluation standards have lagged for AI tools. Despite numerous published AI applications in medicine, only a very small fraction underwent evaluation in dedicated clinical trials.
- Bias: AI may help eliminate bias. However, if it’s built on biased data, it may promote bias. For example, if data are from one ethnic group, the conclusions may not be as accurate for other ethnic groups.
- Ethics: AI algorithms need data, which have to come from somewhere. This can raise issues of ethics and privacy. For example, STAT says Mayo Clinic used de-identified patient data to fuel AI innovation, but the patients were not asked for consent.
- Liability: Since AI applications are still new, little case law exists. An article in Modern Healthcare warns that liability concerns could prevent AI adoption in hospitals.
Healthcare AI Risk Management
The potential of AI is tremendous, but healthcare leaders need to proceed with caution. Regardless of the type of AI application in question, recommended best practices include:
- Vet systems and vendors carefully.
- Use comprehensive contracts that address potential liability scenarios.
- Train healthcare providers to avoid overreliance on technology. AI tools can assist, but physicians must be in charge of decision-making and communication.
- Always check voice-to-text transcription for errors.
- Use AI applications as intended and according to FDA clearance. Strictly follow labeling and vendor instructions to avoid additional liability.
- Monitor and reassess the system’s performance on a regular basis.
- Maintain a full history of changes to your electronic health record and “black box.”
- Thoughtfully examine how AI interaction will appear in the patient record, keeping the 21st Century Cures Act in mind.
- Consider new documentation standards for AI and physician interaction – particularly when the AI recommendation is rejected.
- Promote data that represents populations with accessibility, standardization, and quality.
- Prioritize ethical, equitable, and inclusive medical AI, addressing explicit and implicit bias.
- Contextualize transparency and trust, which means accepting differential needs.
- Focus in the near term on augmented intelligence rather than AI autonomous agents.
- Create and deploy appropriate training and educational programs.
- Leverage frameworks and best practices for learning healthcare systems, human factors, and implementation science.
- Balance innovation with safety through regulation and legislation.
This article was based in part on a Coverys presentation “Risks and Legal Aspects of Artificial Intelligence in Healthcare,” presented by Matthew P. Keris, Esq., and Judy Recker, MHA, RPh, CPHQ, CPHRM.
Copyrighted. No legal or medical advice intended. This post includes general risk management guidelines. Such materials are for informational purposes only and may not reflect the most current legal or medical developments. These informational materials are not intended, and must not be taken, as legal or medical advice on any particular set of facts or circumstances.